The need and importance of data observability
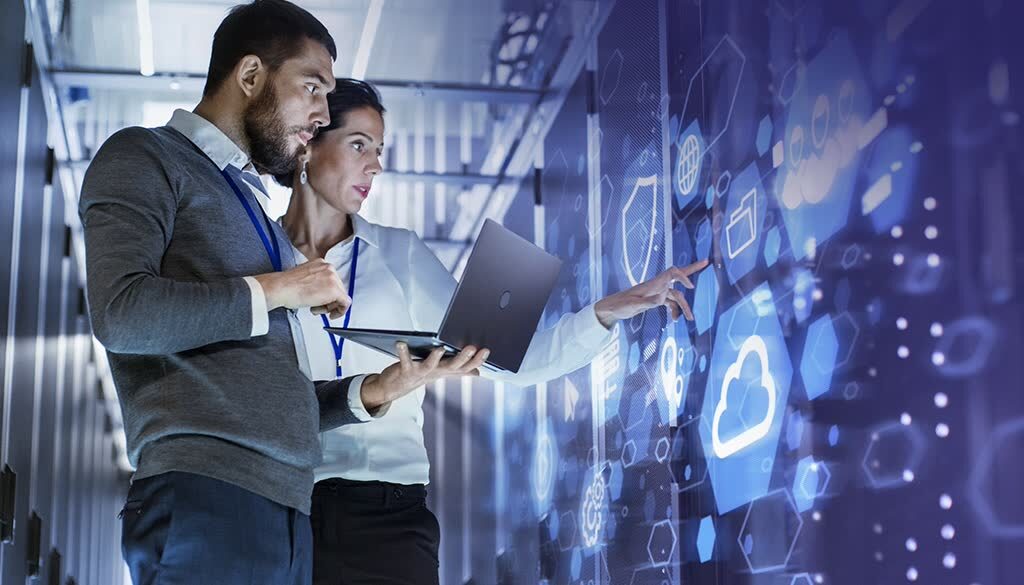
Today, data has become the lifeline of most organizations. Yet, few have complete visibility into their data. As data usage and complexities have grown exponentially, so has the complexity of data systems. The need for Data Observability has never been greater, and the new technology is poised to change the way data scientists, engineers, and analysts work. Let’s examine how this new technology can help organizations.
As industries grow and organizations become colossal in dimensions, there is an increased need to monitor and understand the health of data in its systems, but more importantly, to resolve data issues, if any, in near real time.
This is where ‘Data Observability’ comes in. Data observability helps to identify, troubleshoot, and rectify issues related to data. It is a turning point for industries to have this Data Observability that once was a nice-to-have function, to become an actionable activity.
Data Observability automates data monitoring along the pipelines to facilitate data issues troubleshooting and prevention, so data teams can improve their time to value and scale their productivity.
Data Observability also increases communication across teams by providing evidence-based information on data management ecosystems, while improving trust when it comes to putting applications, models, and reports in production.
Data Observability and Quality of Data
The key to improving trust is the quality of data. Here is where the fundamental difference between data monitoring and data observability is relevant. The difference is the level of context. Monitoring shows the current status of data, while observability gives context and suggestions for solutions. While data monitoring tells you about a problem, observability focuses on providing a proactive approach that can help you prevent problems in the first place. By using data observability techniques, you can ensure the quality of your data, ensuring you get the most value from it.
The first step in building an observability framework is to gather information on the health of your data platform. This can be done by collecting operational data on your system and then analyzing it for potential issues. Data monitoring provides an overall view of the health of your data platform, giving your team visibility into problems across your organization. Further, it provides information on the cause of problems and the downstream impact. Once you have collected and analyzed data, you can use it to design a more reliable and observable system.
As data is always changing and evolving, you need to monitor it regularly. With data observability, you can monitor the state of your data in real time, without having to manually monitor it. A data engineer will also monitor data on its usage and use it to make informed decisions. Data engineers use data observability tools to ensure that their systems operate as expected. The data observability solution should minimize the need for manual monitoring and ensure that compliance and security requirements are met.
The last step of data observability is to standardize the activities that are performed on data. Without visibility of these activities, downstream teams cannot trace problems or make improvements without a good understanding of the upstream processes. A data engineering strategy must have this at its core. The importance of data observability is evident when the following steps are taken:
In order to measure the health of data, the process of production must be reliable. If data integrity is compromised, the business is at risk. Observability tooling should rely on metadata and query logs rather than individual records. This way, it won’t be difficult to monitor data health and improve the quality of analysis. Observability is crucial for the data engineer and data engineering teams. Observability will be more important in the coming decade.
For an organization to be truly data-driven, data observability must be a central part of the overall process. Data observability techniques make data governance strategies and frameworks actionable. Data observability, or “data logging,” is closely related to data governance and reliability. Ultimately, both Data Observability and Data Ops should be incorporated into a data management program. When data quality and consistency are prioritized, data engineering teams are more likely to meet the business’s demands.
What Relevantz Can Do for You
Relevantz helps enterprises get more from their data. With our technology acceleration and data platform services, we build end-to-end data engineering pipelines — covering all six pillars of data engineering — as well as perform modernization, migration, and maintenance of those pipelines to keep the data flowing like it should.
Do you need help with data observability to manage your data better?